AI-driven discovery of novel chemical structures by mass spectrometry
The Zamboni group presents a generally applicable method that enables de novo generation of chemical structures directly from mass spectrometry data. The work was done in collaboration with the group of Prof. Böcker in Jena and was recently published in Nature Methods.
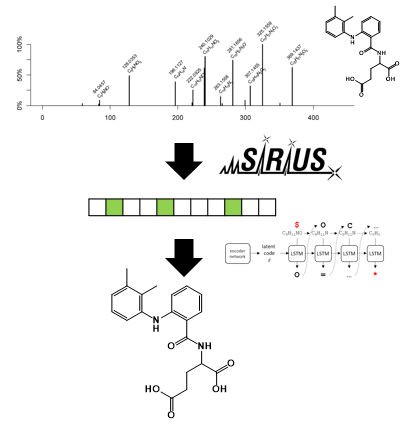
Mass spectrometry is the world-wide predominant technology for detect and quantify chemicals, metabolites, proteins, lipids, or further natural products in all kinds of samples. Further, it supports the identification of unknown molecules by inducing their breakdown – typically by collisions against other molecules - and detection of the resulting fragments: the so-called MS2 spectra. As the MS2 fragments of a molecule depend largely on its structure, the information can be used for structural elucidation.
Even after decades of active research by hundreds of expert groups world-wide, annotating MS2 spectra remains a bottleneck. The current methods for structure elucidation of small molecules rely on finding similarity with spectra obtained with pure standards or simulated from structures listed in databases.
This paper introduces MSNovelist: a generally applicable method for de novo structure generation directly from mass spectra. This was achieved by splitting the underlying problem into two consecutive machine learning tasks. This strategy allowed to overcome the limited amount of data available to train such a deep learning generator. For technical details we refer to the publication and the blog entry that describes the historical developments.
MSNovelist is special for two aspects. First, it is the first of its kind. Second, it is not constrained by any database. This means that it is capable of generating also novel types of molecules, which have never been “conceived” before. Thereby, it opens a new frontier for the discovery of new natural products.
Link to the paper in external page Nature Methods
Link to external page blog post describing the development